Data quality is a major problem in the modern economy because of the increasing reliance on data-driven decision-making. Poor data quality can lead to incorrect business decisions, which can have negative consequences for companies of all sizes.
There are several reasons why companies struggle to ensure the quality of their data across tools including volume of data, speed of data, and growth of data. In most cases, we hear teams seeking tools that help them move faster with data in a confident and reliable way. Let’s navigate each of these points and areas where automation and monitoring of data quality issues can help.
The sheer volume of data that companies generate and collect can make it difficult to manage and maintain. With so much data coming from a wide variety of sources, it can be challenging to ensure that it is accurate, consistent, and up-to-date. This is especially true if you are expected to present this data to people you work within a reliable way, but don’t have the time to look through all the data being presented. This is where automation can help. By implementing tools to check data for issues, accuracy, and getting alerts you can sift through millions of data points.
Data is moving faster than ever across teams and this is causing trust and reliability issues. While the strength of tools to generate and process data has grown, so has keeping up with the demand for high-quality data. The growth of big data and the rise of real-time analytics have put additional pressure on companies to ensure that their data is of the highest quality.
We can’t discuss data quality without talking about silos or places within the organization where data is difficult to view. It happens a lot. Someone comes in and creates a wonderful tool that over time is not maintained in terms of access and this causes troubles down the line when teams across departments want an accurate picture of data. Different teams, departments, and business units may be using different tools and systems to manage their data, which can lead to inconsistencies and errors.
Some flaws we see come in the following areas:
-
One major cause of poor data quality is the amount of manual work in data teams’ workflows.
-
These tasks can include writing boilerplate code, visually checking charts, and reading code to trace dependencies.
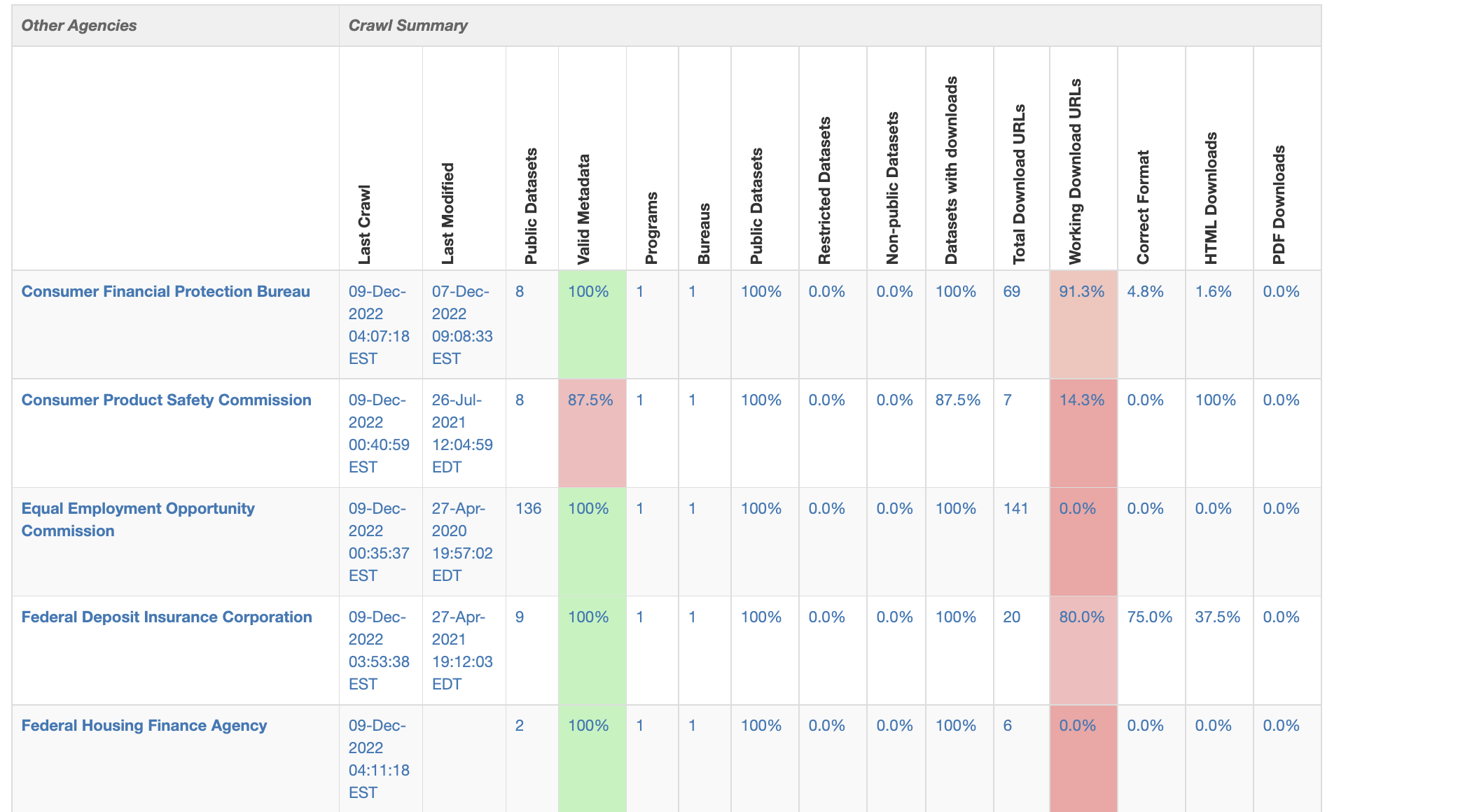
Data is also complex and not every team has a ton of resources dedicated to the skills required to manage data while ensuring quality. The chart above, by Data.Gov reviews a tool that pulls data and checks its formatting using pre-established standards. For the modern data team, this is where new tools come in to help, but most teams want flexibility. They want tools that fit into their existing tools and don’t lock them into one approach, dashboard, or path for exploring their data.
Some approaches we see that work well:
-
Solving data reliability problems at scale involves solving complex problems, but tools that automate this in custom ways drive value.
-
Tools should prevent data quality issues and not add more work to already overloaded data teams. This includes monitoring data issues as they come up.